In this issue:
How Do People Care About Not-COVID?
Weekly COVID Assessment - How are we doing? Where is it bad? Where is it getting worse? How worried should we be?
Deep Dive: Confirmed Cases - Looking at the details and caveats in play when we discuss the metric of “confirmed cases”
No Perfect Metric - A critical review of a Brookings Institute COVID analysis
The Grasshopper and the Ants - This issue’s look at a classic Disney cartoon
Not-Covid? Not Interesting.
It’s odd for me to see Twitter move on from the coronavirus crisis to talk about Mike Flynn or the 2020 presidential election. I don’t know what is going on with any of that and I don’t care to learn. Maybe smart people I trust can fill me in later this summer, but I watch it glide by in the news and I wonder how anyone has the capacity to engage any topic that isn’t this one.
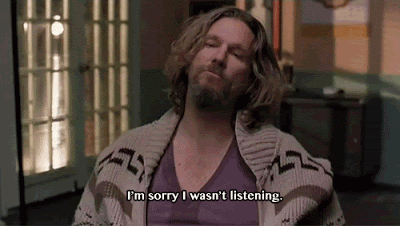
I certainly do not have that kind of energy. The only thing that interests me right now is COVID-19 and the seemingly endless things to learn around it. I struggle even to stay on top of the number of topics that seem to matter in this crisis. Spread, transmission methods, R0, mortality ratios, hospital capacity, excess deaths, the list of things to keep track of goes on and on. And if you *really* want to decide that you’re going to closely follow and comprehensively understand any one of these metrics, you’re going to have to give up being able to carefully follow one of the other ones.
To enhance the complexity of it all, different metrics are more meaningful at points of the crisis. But I’m getting ahead of myself… we’re talk about metrics in a moment. Now it’s time for the…
Weekly COVID Assessment
With months of COVID data behind us, I’m trying to focus on only the more recent data. At the moment, I’m looking at deaths per million residents. For the last week in the US as a whole, that number was 30 deaths per million (dpm) residents.
The worst area continues to be the New England area. While New York (65 dpm) itself has been doing better, New Jersey (134 dpm), Massachusetts (128 dpm), and Connecticut (115 dpm) continue to struggle.
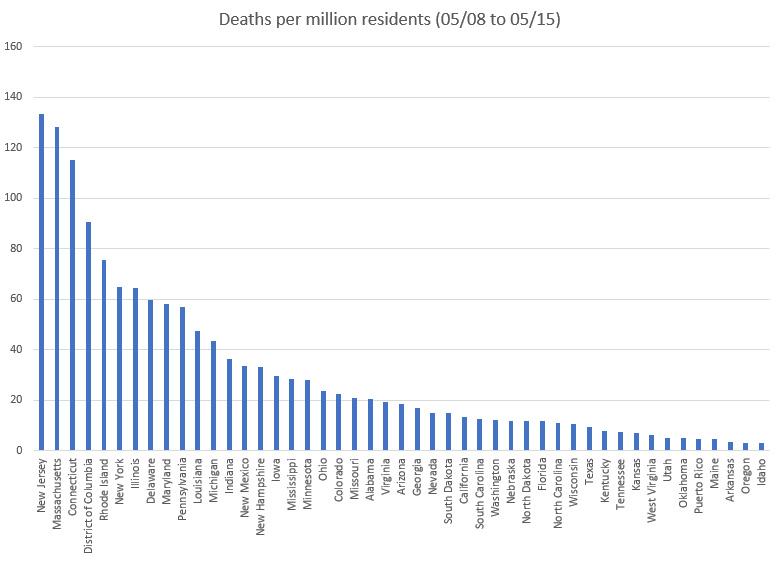
Note: if your state is uncharted here, it is because Hawaii, Montana, Vermont, Wyoming, and Alaska had 0 deaths per million residents.
Many states that have gotten hit hard but, even as they come far down from the worst of it, they are still seeing pretty high death rates. Louisiana is still at 48, Michigan is at 43. That’s better than before, but still not great.
Whenever you see some article slamming a state or region for their decision to open, we need to look at that state’s data and ask the most important question in all of data analysis: “Compared to what?”. If the author gives you an appropriate point of comparison, it’s a good indication that you can trust this person to be honest with you. If they do not, it’s a bad sign and you should be immediately suspicious and careful.
There’s a reason I keep yapping on incessantly about the need for perspective when it comes to assessing a state or region. Looking at just this last week, Texas’s deaths per million is 9. That is one seventh the rate of New York and less than 10% of New Jersey.
Even so, it is is 50% higher that that same number at this time a week ago (6 dpm). That’s not encouraging. We should certainly keep an eye on it. But we are not looking at the kind of acceleration in cases or deaths that we saw in New York in the early stages of this crisis.
It seems enormously likely to me that by the time New York and the surrounding areas reach Texas’s current levels of infection and death, they will be popping champagne and opening up in the way that Texas currently is. I’m not confident enough to call that a prediction, but it’s something to keep an eye on. We tend to gauge the present against the past and we would all be quite happy to see New York hitting Texas-like metrics at this point.
Speaking of metrics, I’m going to sift through some of the common metrics over the next few weeks and talk about what they are useful for, when we should use them and when we be skeptical of their use.
Deep Dive: Confirmed Cases
The metric “confirmed cases” is simply the number of COVID cases confirmed with a medical diagnosis in a given region. At the beginning of the COVID outbreak, this was an extremely important metric to track because it told us how fast the infections were spreading and how widely infected an area is.
Or… it should have told us that. Here in the US we were severely hampered by poor testing early in this crisis. With a properly scaled testing apparatus, it’s likely we could have known the extent of the outbreak in Seattle and New York City at a much earlier point and have moved to lockdown earlier.
From a charting perspective, watching confirmed cases is valuable in the early stage of the crisis and when we’re watching to combat a second wave. In the early stages, we will watch confirmed cases, especially to watch how quickly they double. That is an indication of how fast the infection is spreading and it can be a huge alarm bell that we need to take action immediately to stop an infection that is growing out of control.
However, there are some reasons to be wary of confirmed cases.
You may have seen this chart over the last few weeks. It’s a valuable chart if you’re watching initial infection stages, but I believe this charting style has out-lived its usefulness.
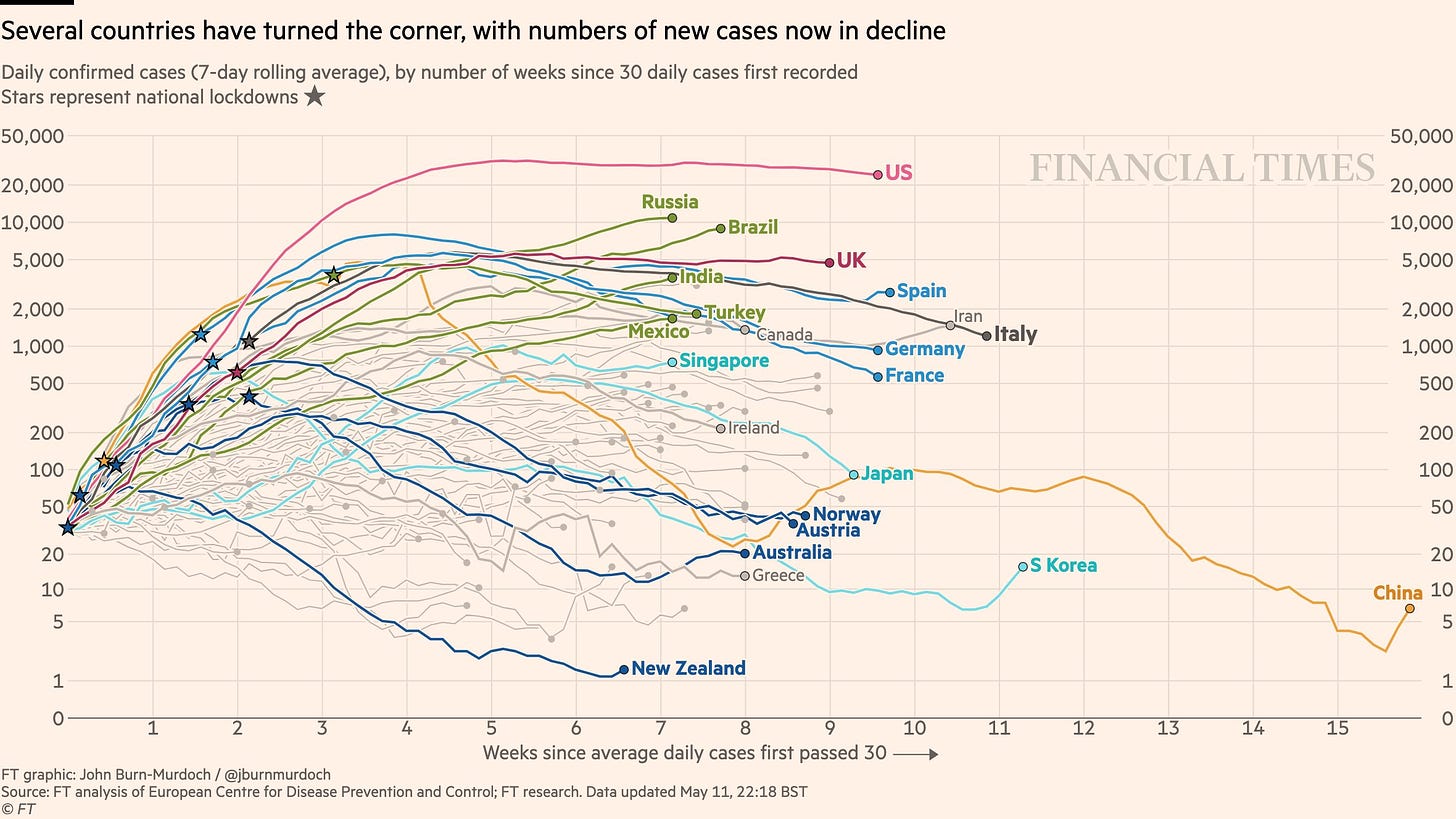
This chart is roughly logarithmic. We use a log chart to understand things that double quickly. You’ll note that, instead of a linear y-axis, this y-axis doubles from 1 to 5 to 10 to 20, 50, 100, 200, 500, and so on.
When an infection starts, it is incredibly important to watch for the case doubling rate (which is what this chart is made for). But that is most important when you’re looking a region, perhaps an urban center or town or any community that lives and works in the same general area. Whether you have a region of 100,000 or a region of ten million, the doubling rate will always start out at the same scale. 10 cases turns into 25 cases, which turns into 50 cases, which turns into 100 cases, etc. Measuring how fast this is happening is a big deal and matters a lot… at the beginning of the crisis.
But once you get past the point of exponential growth, the peaks will be different because different populations will peak at different points. A city of 300,000 will peak at a much lower point than a city of 10 million and it’s not particularly helpful to compare them to each other past the initial point of the infection curve. As we approach the peak, we would probably want to shift into looking at cases per 100,000 people to compare the pandemic severity across different regions.
But we also need to know a little more about the details of case confirmation. We will not catch every case. The confirmed case number is heavily dependent on test availability and reliable reporting structure. On both these things, the US started out poorly but has ramped up substantially. We now have enormous testing capacity and reliable reporting across all states.
This is actually not common. Not all countries report all cases. Not all countries report negative tests. The inconsistencies in this have led many people to draw poor conclusions if they are unfamiliar with the inner workings of data gathering process and assume the data is complete and infallible.
For example, in the US, we have lost about 90 thousand people to this disease among 1.5 million identified cases. That’s 1 death for every 17 cases. Many European countries report a death for every 6-10 cases. This means either this disease is more deadly to European residents (unlikely) or we’re identifying and reporting more infections (likely asymptomatic ones). Anyone who then denigrates the US for having an out-sized number of cases reveals that there is a lot of “under the hood” detail to this metric that they don’t fully understand.
The other reason to be wary of is when someone is talking about confirmed cases but they neglect to note that there is an increase in testing. An increase in testing will, in almost every circumstance, result in an increase in case count. Sean Trende pointed out that when CNN ran with the headline that Texas was seeing a sharp increase in cases, they were giving their viewers a false impression since the case increase was directly related to their intentional and laudable surge in testing.
The surge in testing in the US has been pretty remarkable and we honestly should be proud of ourselves. In fact, our ramp up on testing has been an enormous bright spot in this. We are over 2 million tests a week, which is an excellent capacity for a country of our size. That’s incredible and we should be talking about this remarkable milestone with no small amount of pride.
No Perfect Metric
As the weeks go on, I’ll dig into more of the metrics, what they mean and why we might use them. But I’ve discovered that
there is no perfect metric
we should be very wary of anyone who paints with large brush strokes or tries to use a particular metric to tell a story that dances with partisanship
I was reminded of this as I ran across this article from the normally respectable Brookings Institute.
It’s important to remember that everyone is doing their best, including the authors of this piece. But they’re letting their analysis get in the way of contextually aware thoughtful analysis.
Here is what likely happened, point by point
The authors of this analysis decided that the metric that indicated “high COVID-19 prevalence” was 100 cases per 100,000 residents. I don’t know why they did this and I refuse to guess.
They grabbed the county-by-county COVID case data from the New York Times (here is the raw data file for the more data minded)
They mushed that information against the Census Bureau population data
They barfed out a map that told the story that they wanted to tell… that the COVID crisis is moving from urban centers to more rural centers
They wrote an analysis based on this map without really re-evaluating any of the data or asking any interesting or skeptical questions about what their map told them
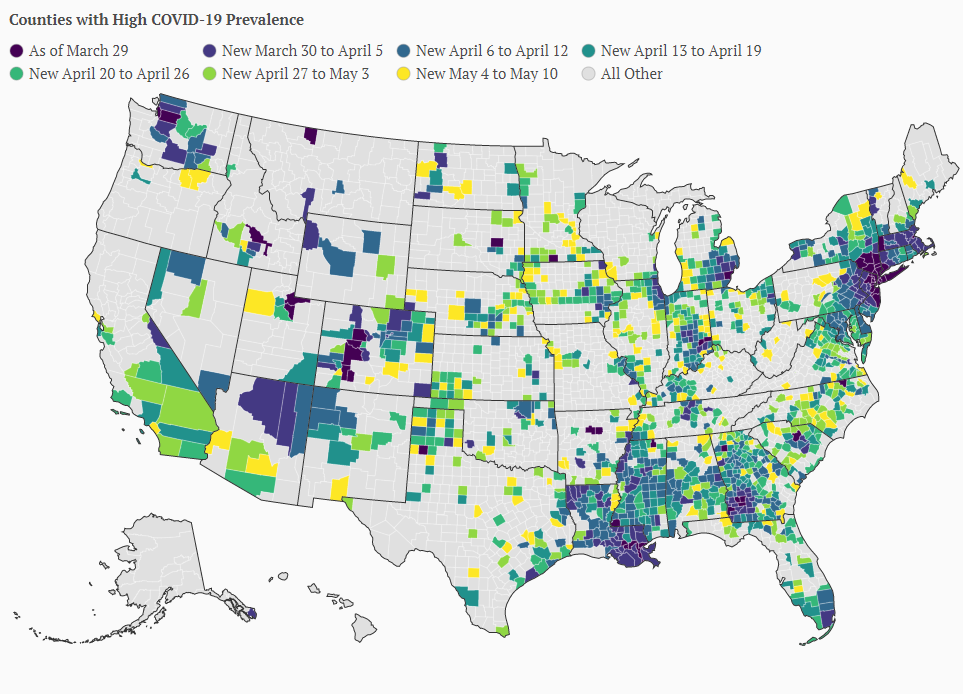
I could poke holes in this analysis… so I will!
The first problem is that I’m not sure where they got this “100 cases per 100,000 residents” metric as the trigger point for “high prevalence” but it does not effectively communicate what a normal reader would consider “high prevalence”. Areas that are considered hot-spots for COVID transmission have rates that are 5 times, 10 times, 20 times that high. Equating them all as “high prevalence” masks the fact that there is an enormous range in this category.
Another issue is that the authors don’t do any kind of time-based analysis. The positive case counts never go down, they only go up. Look at McKenzie county in North Dakota. On April 16th, McKenzie counted their 10th case. Twenty days later, they had identified 3 more cases. Then they identified two more cases in one day (May 7). Those two cases flipped the trigger for the Brookings analysis and they are suddenly “high prevalence” for COVID.
Except they aren’t. Any understanding of McKenzie county would let us know that the blanket application of this metric does not tell a complete story. We would know more about McKenzie county by doing an 15 minute interview with the 2 people who tested positive than by running a nationwide data analysis and coloring their county with the dreaded yellow color based on an arbitrary metric.
The real problem with this analysis isn’t even so much that I can poke holes in it. The problem is that the authors aren’t asking these skeptical questions about their own metrics and analysis and have constructed this narrative that isn’t helping people understand what is going on.
A reader of this analysis will think they know what is happening in rural America. They will be expecting COVID to soon crush these communities due to sparse hospital access. They will think that COVID transmission is not related to population density. They will think that Trump voters are discarding social distancing and sanitation warnings and acting with careless disregard.
We need to take the care and time to curate accurate impressions in this crisis. And we need to be very very skeptical of anyone who is telling a story that has any hint of partisanship in it.
This is not a partisan crisis.
The Grasshopper and the Ants
For this issue, I picked this the Disney short The Grasshopper and the Ants because it inexplicably started showing up in my Disney+ feed as this crisis began in earnest in mid March. I have no idea why, but I have an excellent conspiracy theory that parents who were prepared for the COVID lockdowns started showing their kids this short as a way of explaining why everyone else wasn’t ready.
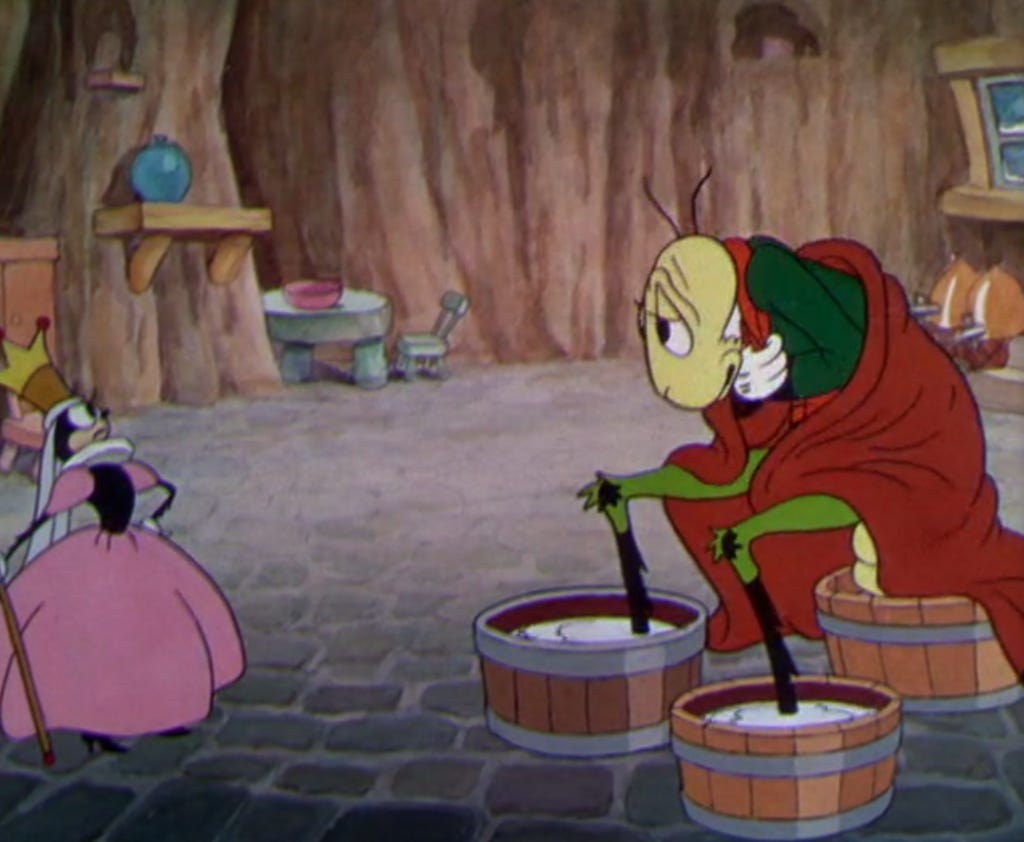
This is a great short and an absolutely perfect connection between the ancient world and our modern one. If you ever heard Goofy singing “oh the world owes me a living”, it is a reference to this short, where Goofy’s voice was first used for a feature character. The concept of the slacker who mocks the industry of the those who prepare in times of plenty is ancient. This fable pre-dates Christ by a good 300 years. But here we are, continuing the ancient tradition through the accident of machine learning and “recommended” watch lists.
In this short, the Grasshopper aims to tempt an ant worker with a life of ease. He even references the Bible with ill intent to encourage sloth and carelessness. The whole thing is a fascinating window into the what society considered to be reckless and foolhardy in the early 1930’s. Example: In several of the Disney shorts of this era (which was solidly in the middle of the Great Depression) the absurd and immature characters are actually quite talented musicians (this short, The Wise Hen, and The Big Bad Wolf all show this). The impression that we get from these shorts is that the slacker losers have had time to practice their music because they aren’t doing anything important.
(I have no moral stake in this concept. I simply find it interesting that musical talent, while clearly valued by Walt Disney himself, is denigrated as a talent of the slothful in many of his shorts.)
Winter comes and the Grasshopper finds himself without food. Here we see one of the earliest examples of a character turning blue to indicate their state of cold.
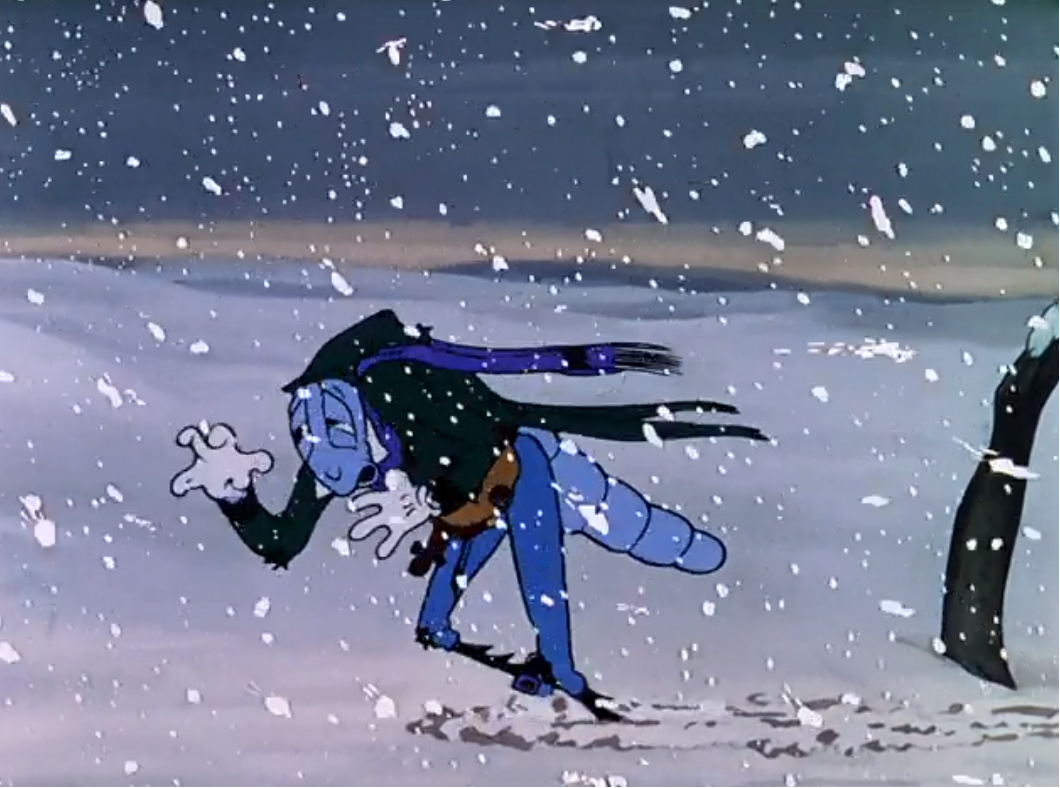
Keep in mind, this is 1934, two years after the debut of the first major color short and 3 years before the first major color feature film (Snow White). Color as an visual medium was still very young and the decision to turn the grasshopper blue was a visual reference to how a person’s lips turn blue during hypothermia. To turn a whole body blue was an innovation of visual media… one that has stayed with us as an unquestioned concept for nearly 100 years.
I think that’s neat.
Anyway, from a moral perspective, Disney is enormously gentle on the grasshopper, granting him shelter and work as an entertainer for the ants during the winter. But his penance comes in the final words of the short, a proclamation that we need in our times of uncertainty.
“You were right and I was wrong”.
"Compared to what?"
Reminds me of Dr. Hans Rosling's book Factfulness. He has a mantra of sorts in there (quote may be inexact), "if someone tries to give you a number, ask for a second. If possible, divide them over another set of numbers." Basically that someone telling you that there are 1,000 cases of X isn't very meaningful. It get's much more useful to know how it compares to a neighbor, perhaps with a different population size, then over time.