I’m never going to get ahead of myself on this and I’m just going to have to accept that.
Things are heating up in just about every state that avoided a first wave. There is reason to hope, but I’m pairing that with a sort of steely determination to be prepared for bad news and difficult numbers. And probably I should be on Twitter less and writing more.
When Should We Be Certain?
Terms and Conditions and Contact Tracing
What Data Do I Use?
Disney Shorts: The Brave Little Taylor
When Should We Be Certain?
One thing I’m always trying to wrestle with is if I’m getting something right or not. I can pull data and make assumptions, but it often takes time, thought, and outside expertise to know something well enough to express an appropriate amount of confidence. I’ve grown to dislike when people dress up a guess as knowledge and I try very hard not to do that myself.
Which makes me think about the last 5 weeks. A month ago, I looked at the data and mentioned that Arizona looks bad, and we should keep an eye on North Carolina. At the time, North Carolina seemed like the most likely candidate for a surge. I’ve been trying to keep up every week with more updates, stay on top of the latest, sift the information as I see it.
But I do want to take a moment to re-stress how uncertain and volatile this data is.
Here are a data set from early May to late June. I remember thinking at the time that it was absolutely remarkable that we have this cluster of states that seem to following an almost identical pattern. California, Georgia, Nevada, and Texas had been extremely close to each other (with Louisiana also very close to them in case counts) and, on June 24th, it looked a lot like they had been on a near-identical slope for almost a month
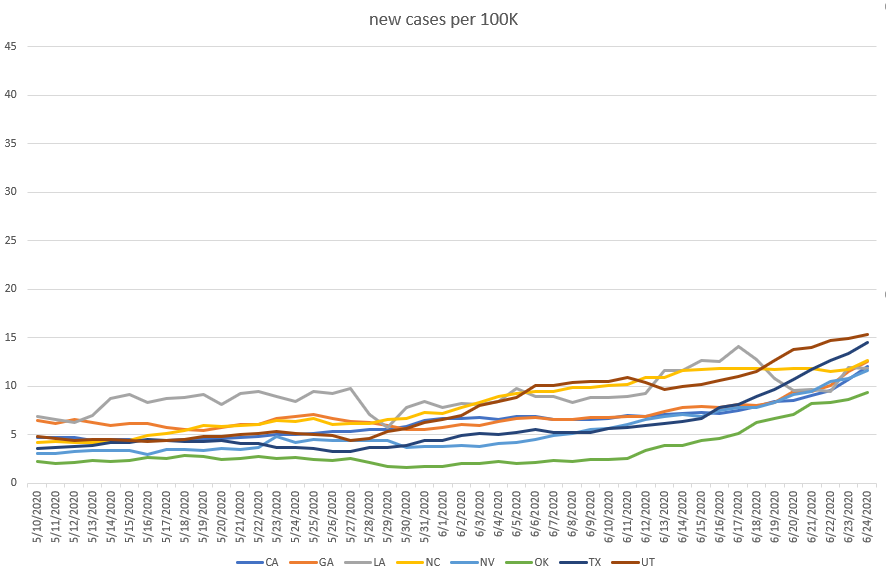
But then something happened. Utah and North Carolina had been higher in case counts, but over the next two weeks they rose much more slowly than the other states in this chart so that a little over 2 weeks later, they are toward the bottom of this group.
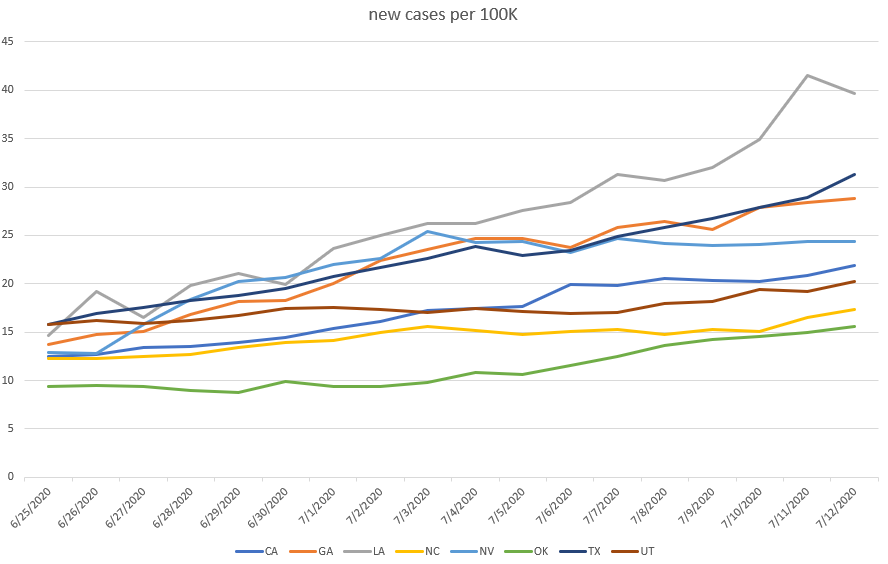
I fully expected these states to stay very close to each other on the trend, but they have diverged. The four states that once looked identical in terms of curve have developed substantially different profiles over the last 2-3 weeks.
I don’t know why and I have found most answers (certainly most simple answers) unsatisfying. As states move past about 10 new cases per day per 100K residents, they seem to hit some point at which they may plateau or they may accelerate and it’s nearly impossible to identify ahead of time which direction a particular state will go. This is why I’m trying to avoid hindsight judgement. Things seem obvious in retrospect but we only ever get to make decisions in light of an unknown future.
No one I know had “air conditioning” on their radar in May when discussing possible reasons for COVID trends. We all assumed that state policies would have much more impact than the weather. With what we know now, it seems that we were wrong. We are, even now, in the middle of being wrong about something else that we likely can’t imagine.
Because we cannot keep out attention focused on more than 2 things at a time, we’re missing the fact that several other states are seeing rising cases in much the same way and to the same levels three weeks ago in the states above. It’s impossible to know how they will turn out right now. Even though North Carolina looked like it was having a bigger surge than Florida or Texas a month ago, their increases have been much lower.
That’s why I keep returning to hope. All this will look obvious in another month but right now we do our best and make our decisions with the information we have. We are not destined to disaster.
Terms And Conditions and Contact Tracing
Back in early May, I was asked by an editor in the American Spectator to author a piece on technology and contact tracing. I said no, because I didn’t know very much about it. Then I read a *lot* of stuff about it and changed my mind. My only disappointment was that it takes forever for things to go into print and I was pretty sure that anything I wrote in May would be obsolete by the time they printed it in July.
And it is, sort of. The magazine is out and no one seems to care about contact tracing right now. But some of what I wrote stands out and I’ve excerpted a bit and encourage you to subscribe to The American Spectator to read the whole thing.
In what I’m going to call “classic epidemiology,” contact tracing is basically just an interview with an infected patient. An interviewer would take the incubation period (between two and fourteen days) and ask the patient where they were during that time. Were they at home? Did they go out to eat? Did they go to church? Did they visit friends? The interviewer would put together a list of people who might have had close contact with the patient while they were contagious and would check up with them. Those contacts would be tested quickly in the early stages of infection and, if they are positive, quarantined…
...
Even in South Korea, the contact tracing interview is the core component of an effective trace. That part isn’t invasive, excessive, or unreasonable. Humans are actually pretty good at talking to other humans and making judgments about what kinds of behaviors might constitute high-risk for transmitting infection. According to an early publication on COVID-19 contact tracing, the infected patient’s GPS data were used only to verify what was said in the interview. It was the human-to-human communication that drove the bulk of the follow-up testing and subsequent quarantines.
…
This requires enormous amounts of trust to be placed in the contract tracing interviewers and the institutions who are managing the tracing process. People have to believe that these institutions are working with clear intention and with respect for individuals and their decision-making processes. And, in return, we need state and local governments to trust their constituents will abide by the issued warnings.
An example of how this trust might work can be seen in Washington state’s plan for reopening dine-in restaurants. Dine-in restaurants will be required to collect diners’ phone numbers and email addresses and record their time of arrival, which can be reviewed as part of the contact tracing process.
I know many of my admirably liberty-minded friends balk at this, but I see this as an exercise in public trust. Nothing stops me from giving them a fake phone number or email address. But I trust them to use this information only as needed. If I test positive for COVID, there’s no reason to lie to the contact tracers and tell them I wasn’t at that restaurant. And if the person across from me tests positive, I would certainly want to know so that I can also be tested.
Most importantly, this kind of manual logging of dining patrons cannot be “flipped on” at a later date. It is not something that is going to linger just because the government likes to track people. It requires trust from the dining patrons, trust from the business owner, trust from the state government. If anyone starts acting to break that trust chain, everyone will suffer. We are interdependent on the goodwill and honesty of all the involved institutions and actors.
Subscribe to read the whole thing, I honestly think it holds up quite well.
What Data Should We Use?
I’m in the process of expanding my data sources to include more and more perspectives, more angles on the data to try to answer harder questions. It is a very big task and it takes a lot of time, even as I’m writing software to try to make it slightly faster.
I know that most people do not subscribe to this newsletter so I can list links to them, but I also think it’s helpful (even if just for myself) to go through and list my data sources, how they are valuable, and for what purpose I would use them.
The Covid Tracking Project - This is my primary data source, I use it to check state-by-state tests, positives, hospitalizations, and deaths. The team working on this is superb, checking every state health department every day to get the best possible data. They even grade state data so you can know which states run a tight ship.
New York Times Covid-19 Data - The New York Times uploads their county-by-county data to github, where you can download it in full. I mostly like it. I have a small gripe with how they count “expected deaths” and “excess deaths” which I think is simplistic, but their county work is great.
Apple Mobility Trends - Apple is aggregating and publishing their mobility data from iPhone directions requests. The idea is that if people are staying home, they aren’t using their Apple Maps app so this is a proxy for how much people are moving around, how serious they are taking quarantine, how careful they are being about going places.
OpenTable State of the Restaurant Industry - OpenTables is tracking seated diners and seated covers. I confess that I’m not sure what the distinction is so I’ve been looking at seated diners. This is limited to restaurants that use OpenTable, but it is still a decent data set for guessing at restaurant attendance, which seems to be one of the higher-risk activities.
JP Morgan’s COVID Compilations - JP Morgan is publishing some really interesting research on a range of important topics, including credit spending, state and country policies, infection tracking, hotspots, potential treatments, and vaccines. They shove all this research into a set of monthly PDF reports that run about 10-15 pages & it’s quite illuminating.
National Institute of Health Blog and Research - I adore Francis Collins, an exceptionally brilliant geneticist and a deeply thoughtful, kind, and dedicated health director. I will not bore you with his credentials, but they are history-making. He runs the NIH and has re-tooled much of the institute to go all out on COVID research. When he writes, I read it. I will also try to pick through NIH papers if for no other reason than to familiarize myself with terminology that helps me understand what is happening now and what to look for next.
There is more out there (excess deaths, case line data, individuals doing daily deep-dives in their area) giving different angles on different things. And the deeper you dive into much of this data (including how it is collected, cleaned, and published) you realize that simply getting the data and showing a chart may obscure some important detail that changes the meaning. I’m trying to keep on top of as much as possible and do a deep dive when it’s important. Thanks for joining me.
Disney Shorts: Brave Little Taylor
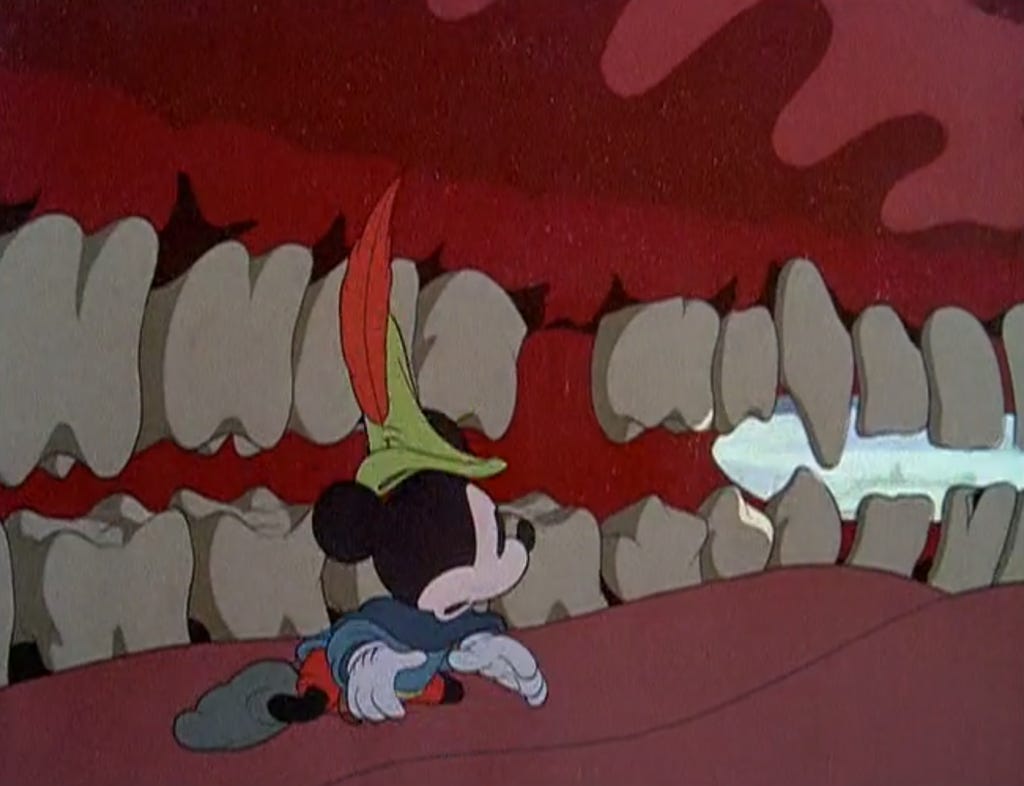
This is one of the greatest Mickey Mouse shorts. Mickey kills 7 flies with one blow of his fly swatter and his story is amplified and misconstrued to turn him into a giant killer. It combines Mickey’s nervous and timid nature with his bravery to rise to a challenge (and his willingness to do anything for Minnie).
It is beautifully animated with lots of wonderful giant gags (smoking a haystack, munching on dozens of pumpkins). It also exemplifies the best of Disney animation in this era, which is an ability to deliver fantastic images and stories in the only format where they could possibly be realized.
In an era of computer generated imagery, we take for granted that filmmakers of previous generations couldn’t show just any story in a live action film. They had severe limitations on what they could technically portray. Disney animation often self-consciously looks for stories in which animation is the ONLY possible option for telling that story. In many cases, what they are animating is something that we wouldn’t be able to effectively replicate outside of animation for the next 70 years.
The scene in which Mickey is inside the giant’s mouth is such a scene. In a scene impossible to comprehend as a live-action film in 1938, the animators deliver stunning imagery imagining what it would be like to be inside a giant’s mouth, with detailed teeth, tongue, and chewing motions. It’s a unique and detailed vision of peril only available through the medium of animation.
Not sure if 10 cases per 100k is a tipping point, or just where you can start seeing the effects of policies and behaviors more readily.
What really matters is the R value at any given time, which is how many other people an infected person infects, on average. The is a function of the policies and behaviors of a given region or population (R0) and the percentage of people susceptible. Let's say both NY and TX implement policies that lead to behaviors that increase R0 to 1.5, say, opening restaurants, but in NY, only 50% of restaurant workers and patrons are still susceptible, but in TX, 97% are, because there was never a real outbreak in TX. Well, in that situation, cases in NY will shrink, and cases in TX will grow exponentially, even though the behavior of the two populations is identical.
So if you have R-values that are pretty-close-to-but-still-above 1.0, and very few cases, you won't see much in the data. At small numbers, daily fluctuations look indistinguishable from random noise. But maybe when case counts get up to 10 per 100k, that low-exponent exponential growth gets easier to see.
Also, on data sources, I find outbreak.info to be very helpful, as it allows you to look at trends by MSA in addition to state and county.